In the global pursuit of net zero emissions, artificial intelligence (AI) has emerged as a transformative force, revolutionizing how industries and organizations approach environmental sustainability. The journey toward AI net zero represents a fundamental shift in how we combat climate change, offering unprecedented opportunities to reduce emissions across every sector of the global economy.
- The Foundation of AI Net Zero: Energy Efficiency Revolution
- Industrial Transformation: AI-Driven Process Optimization
- Carbon Capture and Storage: AI-Enhanced Solutions
- Digital Infrastructure and Environmental Impact
- Climate Monitoring and Verification Systems
- Agricultural Innovation and Emission Reduction
- Corporate Implementation and Future Directions
The Foundation of AI Net Zero: Energy Efficiency Revolution
The cornerstone of achieving net zero emissions through AI lies in its remarkable ability to enhance energy efficiency across all industrial sectors. AI-driven technologies have demonstrated extraordinary capabilities in optimizing energy consumption through sophisticated data analysis and pattern recognition. These systems process vast amounts of operational data to identify inefficiencies that human analysts might miss, leading to substantial energy savings and emissions reductions.
One of the most compelling examples of AI’s impact on energy efficiency comes from the building sector. In a groundbreaking implementation at a Manhattan office building, AI-optimized HVAC systems achieved a 15.8% reduction in energy consumption. This case study demonstrates the practical potential of AI net zero solutions in real-world applications. The success of this implementation has sparked similar initiatives across urban centers globally, as property managers recognize the dual benefits of reduced emissions and lower operational costs.
Smart Grid Innovation: The Neural Network of Clean Energy
AI-powered smart grids are at the forefront of the clean energy revolution, acting as the central nervous system for modern power distribution. By leveraging artificial intelligence, these grids can adapt in real-time to fluctuations in energy supply and demand, making them a crucial component in achieving a sustainable and efficient energy ecosystem.
One of the most significant advancements brought by AI in smart grid technology is its ability to enhance energy efficiency through predictive analytics. Traditional power grids operate on static distribution models, often leading to inefficiencies such as energy overproduction or shortages. AI-driven smart grids, however, utilize machine learning algorithms to continuously analyze consumption patterns, weather conditions, and grid performance. This allows for automated adjustments that optimize energy distribution, ensuring that electricity is directed where it is needed most while minimizing unnecessary waste.
A key challenge in renewable energy integration has always been the unpredictable nature of sources like solar and wind power. Without effective forecasting, grid operators have historically relied on fossil fuel-based backup power to compensate for fluctuations, reducing the overall sustainability of the system. AI has revolutionized this aspect by significantly improving forecasting accuracy. Through the analysis of historical data, weather models, and real-time sensor inputs, AI-powered grids can predict energy generation levels with remarkable precision. This enables better planning, reducing reliance on carbon-based backups and increasing the percentage of renewable energy in the overall energy mix.
Beyond efficiency and sustainability, AI-powered smart grids enhance grid resilience and reliability. They can detect and respond to disruptions, such as power surges, equipment failures, or cyber threats, much faster than traditional systems. AI-driven anomaly detection algorithms identify potential faults before they escalate, allowing for proactive maintenance and reducing downtime. This is particularly valuable in mitigating the impact of extreme weather events, which are becoming more frequent due to climate change.
Additionally, smart grids facilitate demand response mechanisms that empower consumers to participate in energy conservation. AI-driven systems provide real-time insights to households and businesses, allowing them to adjust their energy usage based on peak and off-peak periods. This not only reduces overall grid strain but also helps consumers lower their electricity costs.
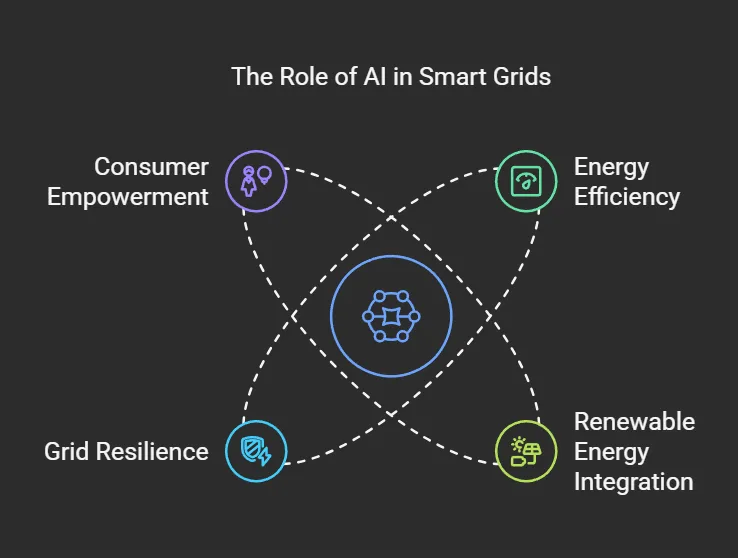
Industrial Transformation: AI-Driven Process Optimization
AI-driven process optimization is playing a pivotal role in transforming the industrial sector toward achieving net-zero emissions. By leveraging advanced machine learning and data analytics, AI is revolutionizing manufacturing and production processes, significantly reducing waste, energy consumption, and carbon emissions.
One of the primary ways AI is accelerating this transition is through predictive maintenance. Traditional industrial systems often suffer from unplanned downtime due to equipment failures, leading to inefficiencies and increased emissions. AI-powered predictive maintenance utilizes real-time sensor data and historical performance metrics to anticipate machinery failures before they occur. This allows manufacturers to schedule maintenance proactively, minimizing production interruptions and reducing energy-intensive breakdowns.
Beyond maintenance, AI optimizes energy usage in industrial operations. Smart algorithms continuously analyze energy consumption patterns, detecting inefficiencies and suggesting real-time adjustments. For example, AI can dynamically regulate heating, cooling, and power distribution across a factory floor, ensuring that energy is used only where and when it is needed. This level of precision significantly lowers emissions, as excess energy consumption is one of the leading contributors to industrial carbon footprints.
Another critical advantage of AI in industrial transformation is process automation and quality control. Advanced AI models monitor every stage of manufacturing, identifying defects or deviations in real-time. This reduces material waste, improves product consistency, and enhances overall efficiency. Additionally, AI-powered robotic systems can streamline assembly lines, optimizing resource allocation and minimizing unnecessary energy use.
AI is also playing a crucial role in supply chain optimization. Industrial supply chains are complex networks with multiple variables influencing efficiency, from raw material procurement to final product distribution. AI-driven logistics systems analyze real-time data to optimize routes, reduce transportation emissions, and prevent supply chain bottlenecks. By minimizing excess inventory and improving delivery efficiency, AI helps lower the carbon footprint associated with material transportation.
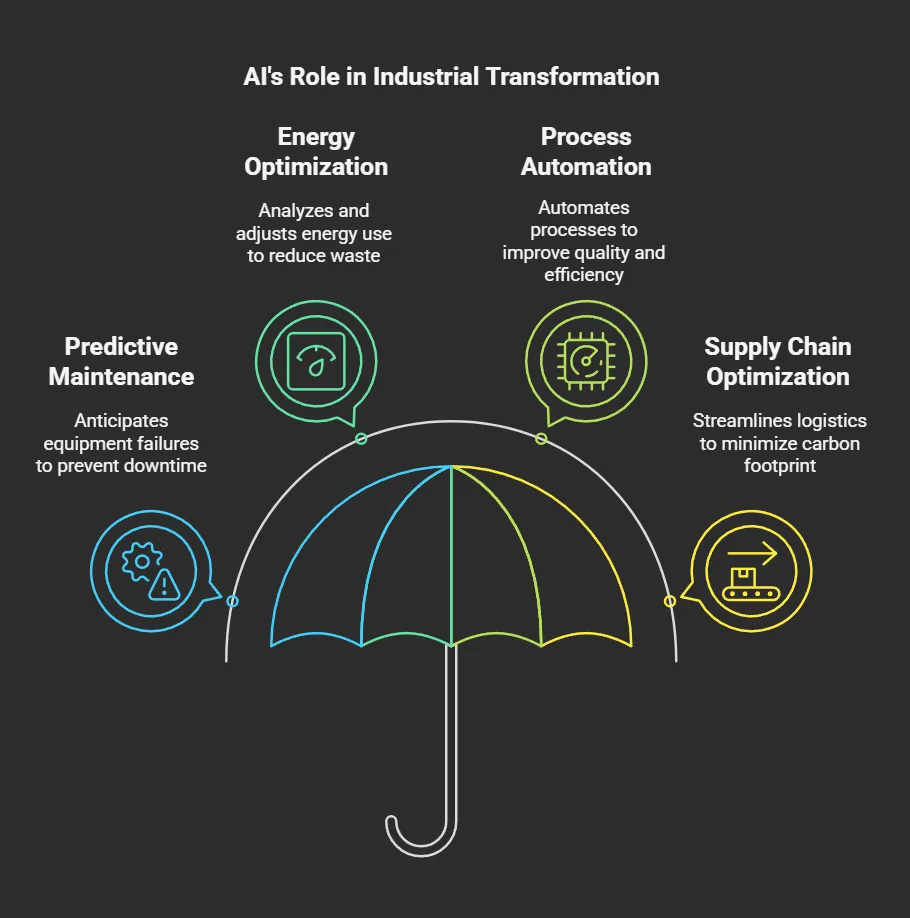
Predictive Maintenance and Resource Optimization
AI-powered predictive maintenance and resource optimization are revolutionizing industrial operations by ensuring peak efficiency and sustainability. Traditional maintenance practices rely on fixed schedules or reactive approaches, leading to unnecessary downtime, increased operational costs, and inefficient resource utilization. AI-driven predictive maintenance overcomes these limitations by using real-time data analytics, machine learning models, and IoT sensors to anticipate equipment failures before they happen.
One of the key benefits of predictive maintenance is its ability to minimize unplanned downtime. Industrial machinery that operates inefficiently due to wear and tear consumes more energy and generates higher emissions. AI systems continuously monitor equipment performance, detecting early signs of degradation such as temperature fluctuations, vibration irregularities, or changes in energy consumption. By identifying potential failures in advance, businesses can perform targeted maintenance at the right time, preventing breakdowns that would otherwise lead to excessive energy waste and production delays.
Beyond individual machine health, AI-driven resource optimization enhances overall operational efficiency. Smart energy management systems adjust power consumption dynamically, ensuring that equipment only runs at necessary levels. In industries with high energy demands, such as manufacturing and logistics, AI algorithms analyze workflow patterns and adjust energy distribution to minimize waste. This leads to lower greenhouse gas emissions and significant cost savings.
AI also plays a crucial role in optimizing material usage. Advanced analytics track inventory levels, raw material consumption, and waste patterns, allowing businesses to fine-tune supply chain logistics. This prevents overproduction, reduces excess resource usage, and ensures that materials are allocated efficiently. By minimizing waste and improving supply chain sustainability, AI contributes to a more circular economy where resources are used more effectively.
In addition to reducing waste and energy consumption, AI-driven systems improve safety and reliability. Predictive analytics can identify hazardous conditions before they lead to accidents, protecting workers and preventing costly operational disruptions. AI-powered monitoring systems detect leaks, overheating, or other environmental risks, ensuring compliance with sustainability and safety regulations.
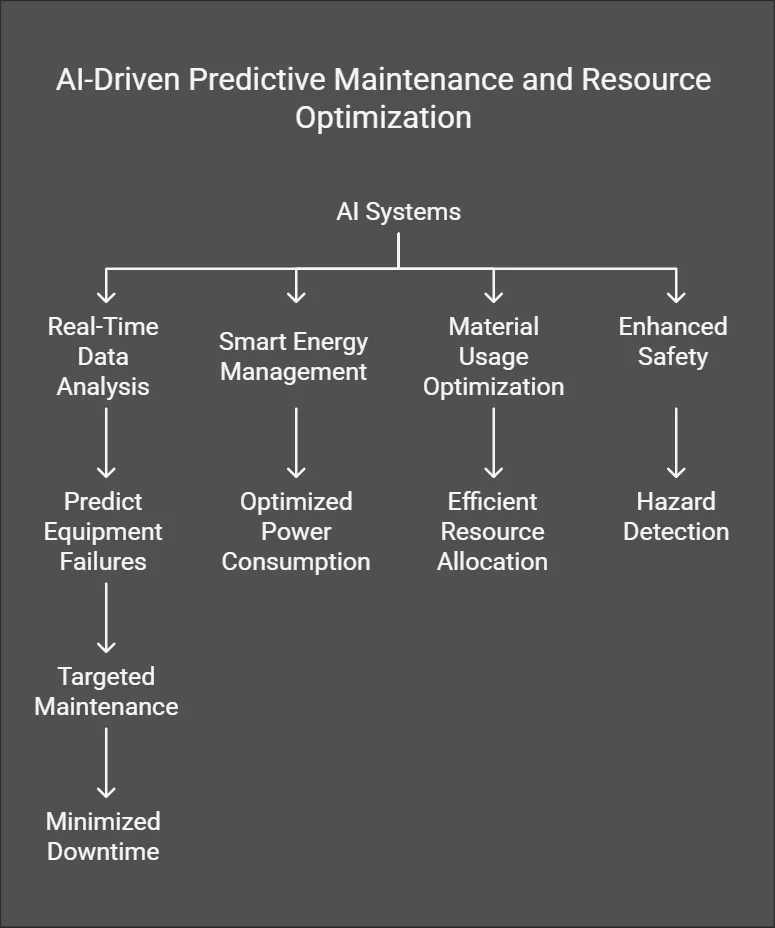
Carbon Capture and Storage: AI-Enhanced Solutions
The role of AI in carbon capture and storage (CCS) technologies represents a critical advancement in the pursuit of net zero emissions. AI systems have revolutionized both the capture and storage phases of CCS operations, improving efficiency while ensuring safety and reliability. Machine learning models continuously analyze operational data to optimize capture rates while minimizing energy consumption, making CCS more economically viable and environmentally effective.
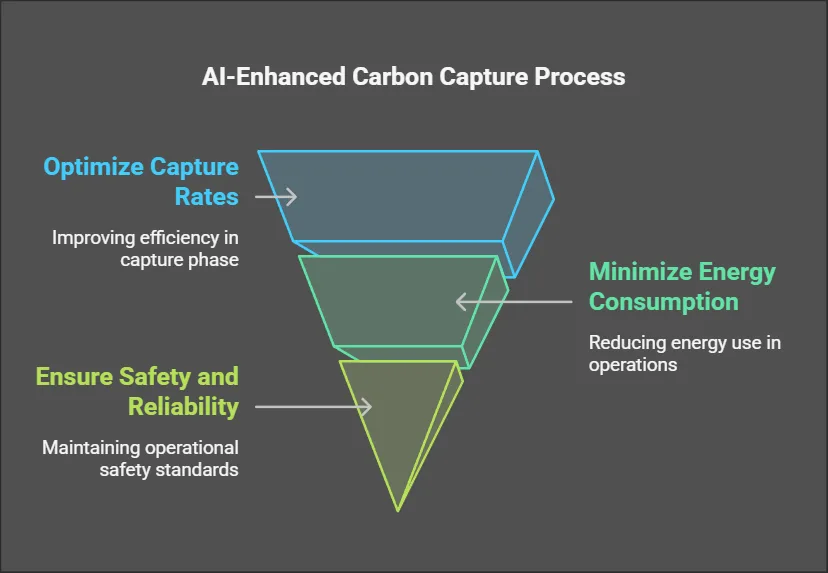
Real-Time Monitoring and Safety Assurance
Advanced AI monitoring systems are revolutionizing the oversight of carbon capture and storage (CCS) facilities by providing continuous, real-time surveillance of stored carbon. These AI-driven systems utilize sophisticated algorithms, machine learning models, and sensor networks to detect potential leaks, structural weaknesses, or operational anomalies, ensuring the integrity and safety of carbon storage sites.
One of the primary challenges in CCS technology has been ensuring that captured carbon remains securely stored over the long term. Even minor leaks can undermine the effectiveness of the entire process, releasing stored CO₂ back into the atmosphere and compromising climate mitigation efforts. AI-powered monitoring addresses this challenge by analyzing data from various sources, including satellite imagery, underground pressure sensors, seismic activity detectors, and gas composition monitors. By processing this vast amount of information in real time, AI can quickly identify irregularities and alert operators to take immediate corrective action before a leak escalates.
Beyond leak detection, AI enhances predictive maintenance for CCS infrastructure. Machine learning models analyze historical data to forecast potential failures in pipelines, injection wells, or geological formations. This proactive approach allows maintenance teams to reinforce vulnerable areas before issues arise, reducing the risk of CO₂ leakage and maintaining the structural integrity of storage sites.
AI-powered monitoring also plays a crucial role in improving transparency and regulatory compliance. Governments and environmental agencies require rigorous oversight of CCS projects to ensure they meet sustainability standards. AI systems can generate detailed reports on storage performance, CO₂ retention rates, and environmental impacts, providing verifiable data that builds public and governmental trust in CCS as a viable solution for reducing greenhouse gas emissions.
Moreover, AI facilitates enhanced site selection for new CCS facilities. By analyzing geological data, historical storage performance, and environmental risk factors, AI models can help identify optimal locations for carbon sequestration. This ensures that new storage sites have the highest probability of long-term success, minimizing the risk of leakage and maximizing storage efficiency.
As CCS technologies continue to develop, AI will remain an integral component in ensuring their reliability and effectiveness. The combination of real-time monitoring, predictive analytics, and automated response systems strengthens the viability of carbon capture and storage as a key tool in the global transition to net-zero emissions. By leveraging AI-driven oversight, CCS facilities can operate with greater security, efficiency, and public confidence, making them a cornerstone of sustainable climate strategies.
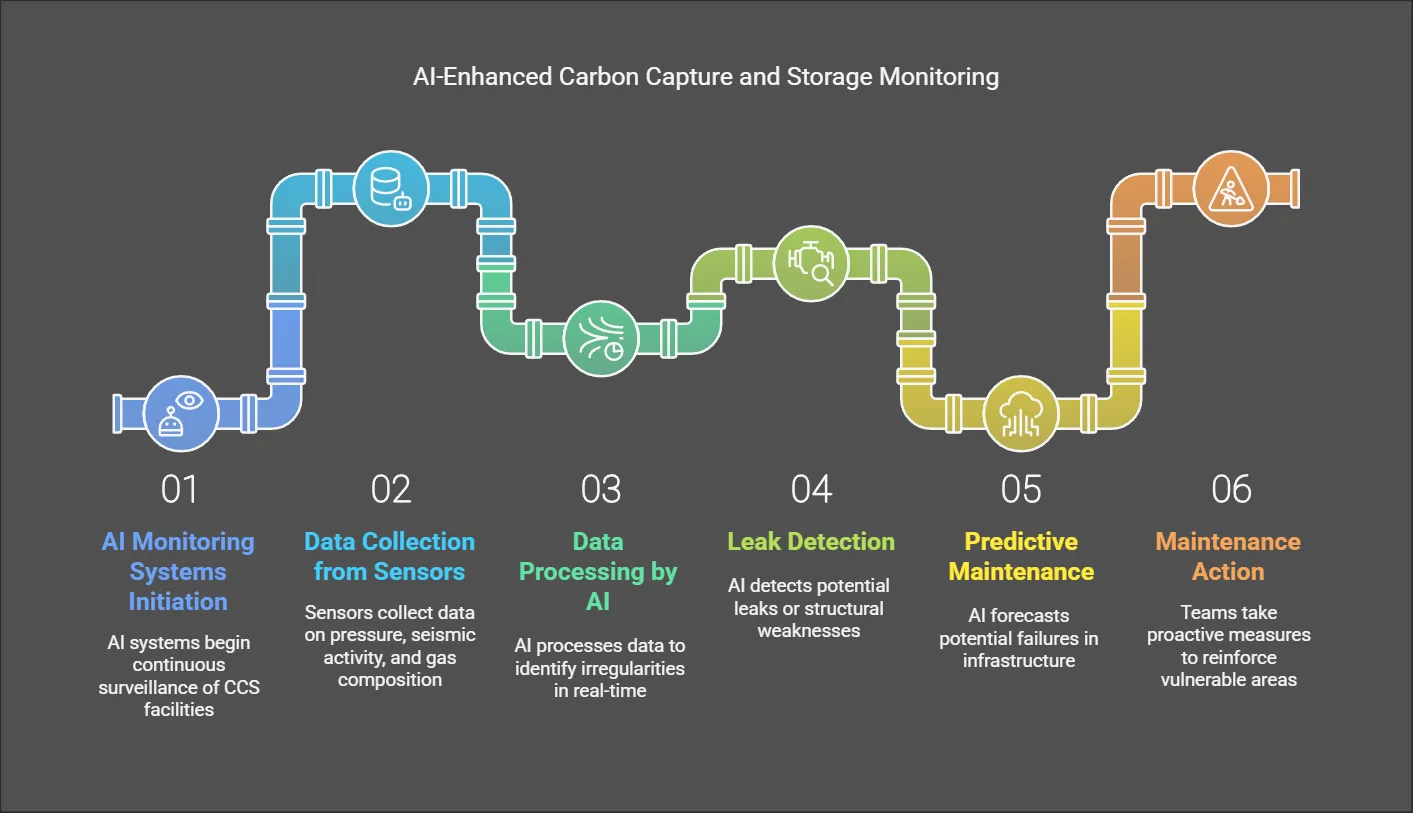
Digital Infrastructure and Environmental Impact
While AI plays a crucial role in driving the global transition to net-zero emissions, the technology sector itself has been actively working to reduce its own environmental footprint. The rapid growth of AI applications, particularly large-scale machine learning models, has raised concerns about their energy consumption and carbon emissions. In response, researchers and tech companies are developing energy-efficient AI models that maintain high performance while drastically reducing resource usage.
A key breakthrough in this area is the optimization of AI algorithms to run with lower computational requirements. Traditional deep learning models, especially those used in natural language processing and computer vision, demand extensive processing power from data centers, leading to high electricity consumption and substantial carbon emissions. New advancements, such as model pruning, quantization, and knowledge distillation, enable AI models to operate more efficiently without compromising accuracy. These techniques reduce the number of active parameters in a neural network, lowering energy demand while maintaining robust functionality.
One of the most notable achievements in AI energy efficiency comes from DeepSeek, a Chinese AI application that has significantly outperformed traditional models in sustainability metrics. DeepSeek has demonstrated a 90% reduction in energy consumption and a 92% decrease in carbon emissions compared to conventional AI frameworks. This efficiency gain is attributed to optimized model architecture, advanced hardware utilization, and intelligent workload distribution across low-power computing environments.
Another major innovation is the shift toward AI-driven sustainability in data centers. Tech companies are increasingly implementing AI-based energy management systems to optimize server loads, cooling mechanisms, and power distribution. By dynamically adjusting computing resources in response to demand fluctuations, AI helps reduce energy waste and improve the overall sustainability of cloud computing infrastructure. Some organizations are also investing in renewable energy-powered data centers, further lowering the carbon footprint of AI operations.
The push for sustainable AI extends beyond hardware efficiency to software-level optimizations. AI researchers are exploring low-power training techniques that require fewer iterations, reducing the time and energy needed to develop new models. Additionally, federated learning, a decentralized AI training approach, minimizes data transfer between devices and central servers, further cutting down on energy consumption.
Sustainable Computing and Data Center Optimization
AI-driven optimization of data center operations is playing a critical role in reducing the environmental impact of digital infrastructure. As the demand for cloud computing, machine learning, and high-performance computing continues to rise, data centers have become one of the largest consumers of electricity worldwide. AI-powered management systems are now essential in ensuring these facilities operate efficiently while aligning with net-zero emission goals.
One of the most impactful applications of AI in data centers is in optimizing cooling infrastructure. Cooling systems account for a significant portion of a data center’s total energy consumption, as servers generate substantial heat during operation. Traditional cooling methods often operate at fixed power levels, leading to unnecessary energy expenditure. AI-driven thermal management systems, however, continuously analyze temperature data, humidity levels, and server workloads to dynamically adjust cooling mechanisms in real time. This allows for precise temperature regulation, reducing energy waste while preventing overheating.
AI also enhances workload distribution, ensuring that computing tasks are allocated efficiently across servers. Machine learning algorithms monitor data center activity and predict usage patterns, shifting workloads to servers with lower energy demand or redistributing them during off-peak hours to optimize power consumption. Some AI-driven systems even reroute workloads to regions where renewable energy is more available, further minimizing carbon emissions.
Energy management is another area where AI is driving significant improvements. By integrating real-time monitoring with predictive analytics, AI helps data centers maximize the use of renewable energy sources while reducing reliance on fossil-fuel-powered grids. Smart energy scheduling enables operators to align power-intensive tasks with periods of peak renewable energy generation, such as during daylight hours for solar-powered facilities.
AI also plays a vital role in preventive maintenance, reducing the likelihood of unexpected failures that could lead to costly downtime and increased energy use. By analyzing historical data and sensor readings, AI can detect anomalies in server performance, power supply fluctuations, or cooling inefficiencies before they escalate into major issues. This predictive capability extends the lifespan of critical hardware while maintaining operational stability.
Leading technology companies are increasingly deploying AI-driven carbon tracking and reporting tools, ensuring transparency in data center emissions. These systems provide insights into power usage effectiveness (PUE), carbon intensity metrics, and overall sustainability performance. As a result, organizations can make data-driven decisions to further refine their energy strategies and align with net-zero commitments.
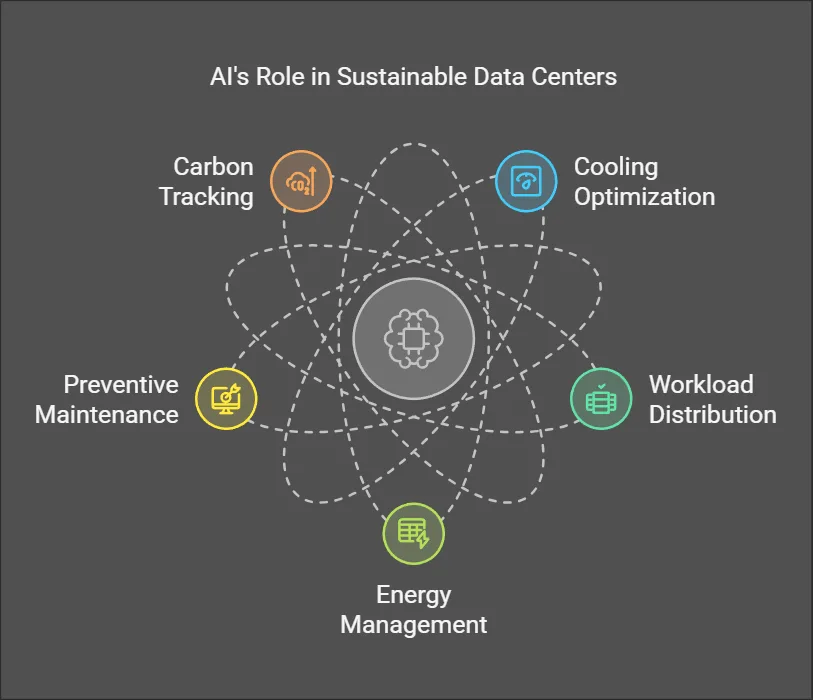
Climate Monitoring and Verification Systems
The development of AI-powered monitoring systems is a cornerstone of the net-zero initiative, providing real-time insights into global greenhouse gas emissions with unparalleled precision. Traditional methods of tracking emissions often rely on self-reported data, which can be incomplete or inaccurate. AI-driven solutions, however, leverage vast datasets from satellites, remote sensors, and ground-based monitoring stations to deliver objective, high-resolution assessments of emissions across industries and geographic regions.
One of the most groundbreaking applications of AI in environmental monitoring is the Climate TRACE initiative. This AI-powered system aggregates and analyzes satellite imagery, sensor data, and machine learning algorithms to create a near-real-time inventory of global greenhouse gas emissions. Unlike conventional reporting methods, which often suffer from long delays and underreporting, Climate TRACE provides independent, transparent, and frequently updated emissions data across sectors such as energy, transportation, manufacturing, and agriculture.
AI’s ability to process vast amounts of data in real-time allows for precise tracking of emissions sources, down to specific industrial facilities or transportation networks. Machine learning models analyze infrared and spectral imaging from satellites to detect methane leaks, carbon dioxide emissions, and other pollutants, making it possible to pinpoint and address problem areas before they escalate. This level of detail is particularly critical for industries that have historically been difficult to monitor, such as maritime shipping and deforestation activities.
Beyond emissions tracking, AI-driven environmental monitoring systems enhance policy enforcement and accountability. Governments and regulatory bodies can use Climate TRACE and similar platforms to verify compliance with climate agreements, ensuring that corporations and nations meet their reduction targets. By eliminating reliance on self-reported data, AI strengthens transparency and empowers policymakers to make informed, data-driven decisions.
The integration of AI into climate monitoring also extends to predictive analytics. By analyzing historical trends and current emissions data, AI can forecast future environmental impacts, allowing decision-makers to implement proactive mitigation strategies rather than reactive measures. This capability is especially valuable in anticipating climate risks, such as extreme weather events or shifting carbon sink dynamics in forests and oceans.
Agricultural Innovation and Emission Reduction
The transformation of the agricultural sector through AI technologies highlights the vast potential of AI-driven solutions in achieving net-zero emissions. Traditional farming methods often lead to excessive resource consumption, including water, fertilizers, and pesticides, which contribute to environmental degradation and greenhouse gas emissions. AI-powered precision farming techniques have revolutionized these practices by optimizing resource allocation, minimizing waste, and enhancing productivity.
One of the most significant advancements in AI-driven agriculture is the use of machine learning algorithms to analyze soil health and crop data. AI-powered sensors and satellite imagery provide real-time insights into soil moisture levels, nutrient composition, and crop growth patterns. By processing this data, AI systems can determine the precise amount of water, fertilizers, and pesticides required for each section of a field, reducing excess usage that would otherwise contribute to environmental pollution and carbon emissions.
AI-driven irrigation systems play a crucial role in water conservation. These systems assess real-time weather conditions, soil moisture, and crop requirements to deliver water only when and where it is needed, significantly reducing water waste. Advanced AI models can even predict drought conditions and recommend proactive irrigation strategies, ensuring long-term sustainability without depleting natural resources.
Another major innovation in AI-powered agriculture is automated pest and disease detection. Machine vision and deep learning models analyze images from drones and on-the-ground cameras to identify early signs of pest infestations or crop diseases. Farmers can take targeted action before the problem spreads, reducing the need for widespread pesticide use, which contributes to greenhouse gas emissions and soil degradation.
AI also enhances farm equipment efficiency by integrating autonomous machinery that operates with minimal fuel consumption. AI-powered tractors and harvesting robots can optimize routes and adjust power usage dynamically, reducing energy consumption while maintaining high productivity. Some of these machines are even transitioning to electric and renewable-powered alternatives, further reducing emissions.
Supply chain optimization is another critical aspect of AI’s impact on agriculture. AI-driven forecasting models analyze market trends, weather conditions, and storage logistics to help farmers reduce food waste and transportation emissions. By predicting demand more accurately, farmers can plan harvests efficiently, ensuring that produce reaches markets at the right time while minimizing spoilage and unnecessary transportation.
Looking ahead, AI-driven regenerative farming techniques, carbon sequestration monitoring, and sustainable livestock management will further enhance agriculture’s contribution to net-zero goals. AI-powered carbon tracking systems will allow farmers to measure their carbon footprints accurately, enabling them to adopt low-emission practices. By integrating AI at every stage of the agricultural process, from soil analysis to market distribution, the industry can achieve greater efficiency, lower emissions, and long-term sustainability while maintaining or even increasing crop yields.
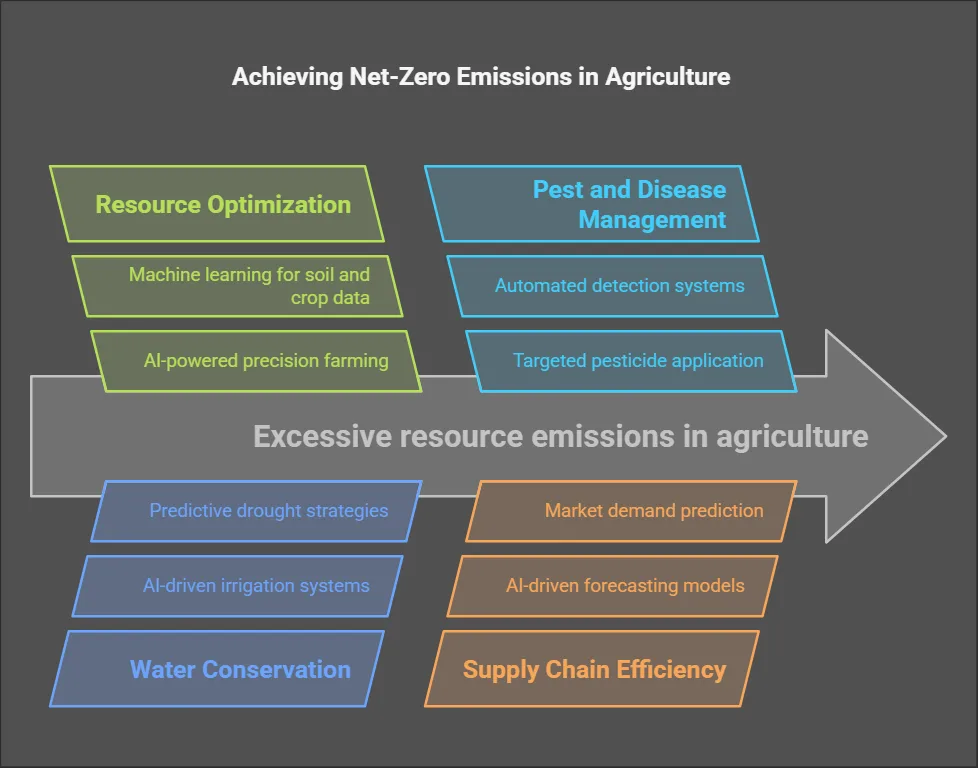
Livestock Management and Methane Reduction
AI-driven livestock management has revolutionized the agricultural sector by introducing advanced monitoring systems that track animal health, behavior, and environmental conditions in real time. These innovations play a crucial role in enhancing efficiency, reducing waste, and addressing one of agriculture’s most significant sources of greenhouse gas emissions—methane production from livestock.
One of the key applications of AI in livestock management is real-time health monitoring. Traditional methods of disease detection in animals rely on visual inspections and periodic veterinary visits, often leading to delayed interventions. AI-powered wearable sensors and computer vision systems continuously monitor vital signs, movement patterns, and feeding behaviors, detecting early signs of illness or stress. This allows farmers to take preventive action before conditions worsen, reducing the need for antibiotics and minimizing production losses.
Another critical AI-driven innovation is feed optimization, which directly impacts methane emissions. AI algorithms analyze individual animals’ nutritional needs based on factors such as age, weight, and metabolic rate. By tailoring feed composition to maximize digestion efficiency, AI reduces the amount of undigested food that leads to excessive methane production. Some systems also suggest alternative low-emission feed formulations, such as seaweed-based diets, which have been shown to significantly reduce methane emissions in cattle.
AI also plays a role in precision breeding programs, where genetic data analysis helps identify livestock with naturally lower methane emissions and higher feed conversion efficiency. By selectively breeding animals with these traits, AI contributes to long-term sustainability improvements in livestock production.
In addition to reducing emissions, AI enhances herd management and resource efficiency. AI-powered drones and smart surveillance cameras provide real-time tracking of animal movement and grazing patterns, ensuring that livestock are evenly distributed across grazing fields. This prevents overgrazing, preserves soil health, and improves carbon sequestration in grasslands—an essential aspect of mitigating climate change.
AI-driven waste management systems also contribute to emission reductions. Machine learning models optimize manure management by predicting the best times and methods for waste processing, reducing methane release from decomposing organic matter. Some AI-powered systems even integrate with biogas generation units, converting livestock waste into renewable energy, further lowering the farm’s carbon footprint.
By harnessing AI for precision livestock management, the agricultural industry is making significant strides toward reducing methane emissions, improving efficiency, and contributing to global net-zero goals. These innovations ensure that livestock farming remains both productive and environmentally responsible.
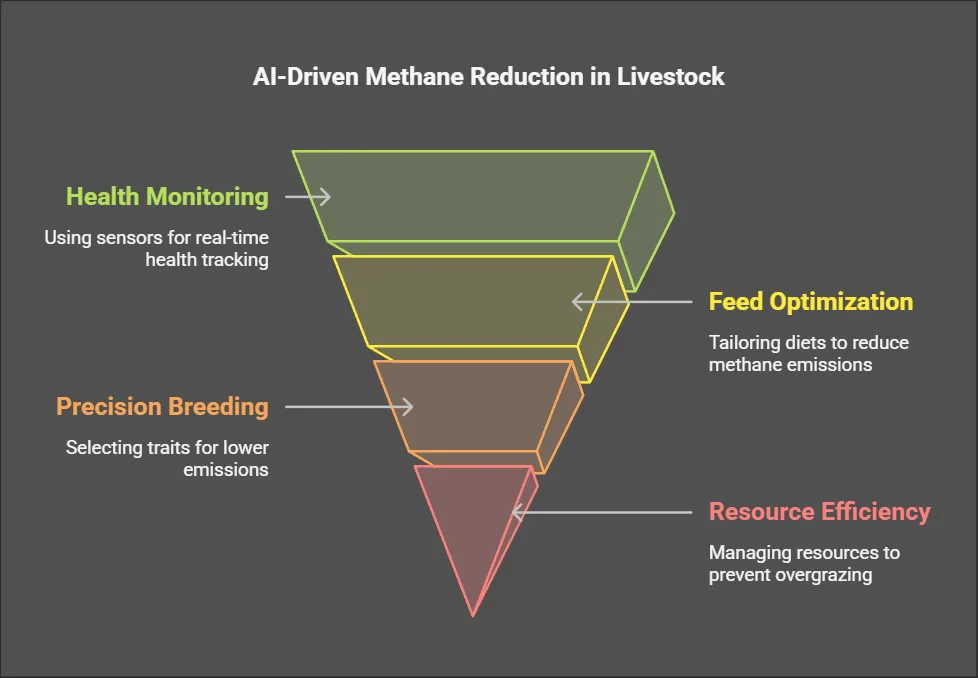
Corporate Implementation and Future Directions
The corporate sector’s adoption of AI net zero solutions continues to accelerate, driven by both environmental responsibility and economic benefits. Advanced carbon footprint analytics powered by AI enable companies to monitor and predict emissions across their entire value chains, facilitating more effective sustainability strategies.
Supply Chain Optimization and Emission Reduction
AI-driven supply chain optimization represents a crucial frontier in corporate emission reduction efforts. These systems analyze complex supply chain networks to identify opportunities for emission reduction while maintaining operational efficiency. The resulting optimizations often deliver both environmental and economic benefits, creating a compelling business case for AI net zero initiatives.
The integration of AI technologies in the pursuit of net zero emissions represents a paradigm shift in environmental protection and sustainability. As these technologies continue to evolve, their potential to accelerate emission reduction efforts grows increasingly significant. The success stories and innovations detailed in this analysis demonstrate that AI net zero solutions are not just theoretical possibilities but practical, implementable strategies for addressing climate change.
The path to net zero emissions requires continued innovation in AI technologies, coupled with thoughtful implementation and policy support. As organizations and governments worldwide embrace these solutions, the potential for achieving ambitious climate goals becomes increasingly realistic. The future of environmental sustainability will undoubtedly be shaped by our ability to leverage AI effectively in the pursuit of a net zero world.
How does AI help integrate renewable energy into smart grids?
AI enhances renewable energy integration by using predictive analytics to forecast solar and wind power generation based on weather patterns, historical data, and real-time sensor inputs. This allows grid operators to optimize energy distribution, balance supply and demand dynamically, and reduce reliance on fossil fuel backup systems, ensuring a more stable and efficient power grid.
How does AI improve industrial sustainability and reduce emissions?
AI-driven process optimization helps industries reduce carbon emissions by improving efficiency in manufacturing, logistics, and energy usage. Predictive maintenance prevents equipment failures that lead to excessive energy consumption, while AI-powered automation reduces waste in production lines. AI also optimizes supply chains, cutting transportation emissions by improving route efficiency and reducing excess inventory.
What role does AI play in carbon capture and storage (CCS) technology?
AI-powered monitoring systems track CO₂ storage sites using satellite imagery, remote sensors, and machine learning algorithms to detect leaks, structural weaknesses, or operational inefficiencies in real time. By improving predictive maintenance and providing transparent emissions tracking, AI ensures the long-term security of stored carbon and strengthens public confidence in CCS as a viable solution for achieving net-zero emissions.
How does AI contribute to sustainable agriculture and livestock management?
AI-driven precision farming optimizes resource usage by analyzing soil and crop data, reducing water consumption, fertilizer overuse, and pesticide application. In livestock management, AI-powered monitoring systems track animal health, optimize feeding to reduce methane emissions, and improve herd management through smart surveillance. AI also aids in sustainable waste processing, converting livestock manure into renewable energy.
How is AI reducing the environmental impact of data centers?
AI optimizes data center operations by managing cooling systems, workload distribution, and energy consumption in real time. It predicts and adjusts power usage dynamically, ensuring energy is allocated efficiently and reducing electricity waste. AI also integrates with renewable energy sources, schedules power-intensive tasks during low-carbon energy availability, and improves hardware lifespan through predictive maintenance, significantly cutting carbon emissions from digital infrastructure.