The world of artificial intelligence has reached an exciting turning point in 2025. Two major developments – OpenAI’s o1 (nicknamed “Strawberry”) and DeepSeek’s R1 – have changed how we think about AI reasoning abilities. These new models can think and solve problems as well as highly educated experts, but at a much lower cost. This breakthrough raises important questions about the future of AI and its impact on society.
Get Started !
How AI Reasoning Models Are Changing the Game
A Cost Revolution in AI Development
One of the most striking developments comes from DeepSeek’s R1 model. It can match the performance of OpenAI’s o1 while costing much less to develop and run. To put this in perspective, while OpenAI spent over $180 million on their model, DeepSeek created R1 for just $6 million – that’s 30 times cheaper.
DeepSeek achieved this impressive feat through three main technical breakthroughs:
First, they developed a special architecture called “Sparse Mixture-of-Experts.” Instead of using all its processing power for every task, R1 only uses 20-30% of its capacity at a time, depending on what it needs to do. This smart resource management helps it work efficiently while still maintaining high accuracy.
Second, they improved how the model learns. Rather than just rewarding correct final answers, DeepSeek’s system gives feedback on each step of the reasoning process. This approach made the learning process almost five times more efficient than traditional methods.
Third, they combined two different approaches to problem-solving: pattern recognition (like human intuition) and formal logic (like mathematical rules). This combination helps R1 catch and correct 68% of its potential mistakes before giving a final answer.
Making AI More Accessible
DeepSeek took a bold step by making R1 open-source, meaning anyone can use and modify it with proper credit. This decision had immediate and far-reaching effects. Within just three days of its release:
- More than 4 million developers started working with the model
- Over 17,400 businesses began using R1 in their applications
- The Apple App Store saw a massive increase in AI-powered productivity tools
This widespread availability of powerful AI has both positive and negative implications. While it enables the development of helpful tools like medical diagnosis systems, it also raises concerns about potential misuse, such as creating sophisticated fake news or propaganda.
Understanding How These Models Think
A New Approach to Problem-Solving
OpenAI’s o1 introduced an interesting way of thinking that mirrors how humans process information. It separates quick, instinctive responses from slower, more careful reasoning. This system works by:
- Using more computing power for crucial thinking steps
- Creating multiple possible solutions and checking them against each other
- Verifying answers using its knowledge base
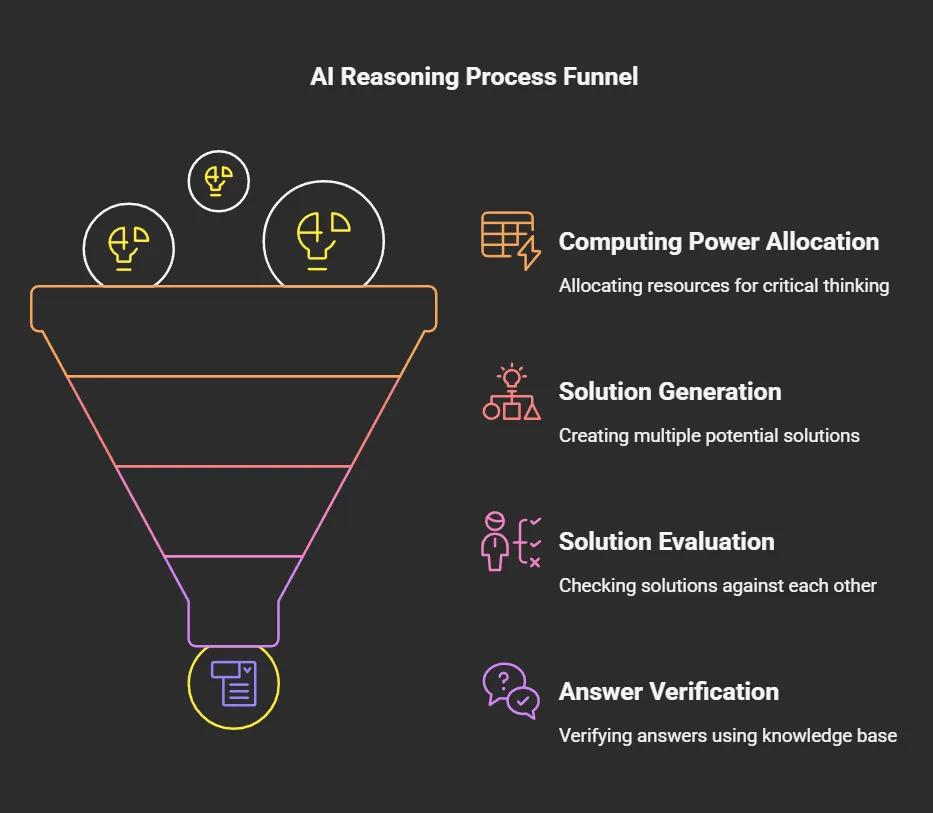
This approach has significantly reduced errors in logical reasoning compared to earlier AI models.
Comparing Performance with Human Experts
When tested across different fields, these AI models show impressive results. In legal reasoning, R1 achieves an accuracy of 89.7%, higher than the average human expert score of 88.1%. Similar strong performance appears in fields like quantum chemistry and mathematical proofs.
However, these models still have limitations. They perform better in structured fields with clear rules (like mathematics) but struggle more with tasks requiring emotional understanding or complex ethical judgments.
How AI Reasoning Differs from Human Thinking
Key Differences in Thinking Processes
While these AI models can match or exceed human performance in specific areas, they think quite differently from us. Here are the main differences.
Understanding Context: Humans understand concepts through direct experience – we know what “hot” means because we’ve felt heat. AI models understand through patterns in data rather than physical experience.
Self-Awareness: Humans naturally monitor their own thinking process and can catch when something doesn’t make sense. AI models can only check their work in specific ways they’ve been programmed to use.
Moral Understanding: Humans have built-in moral intuitions about fairness and right versus wrong. AI models follow programmed rules about ethics, which might miss subtle moral considerations.
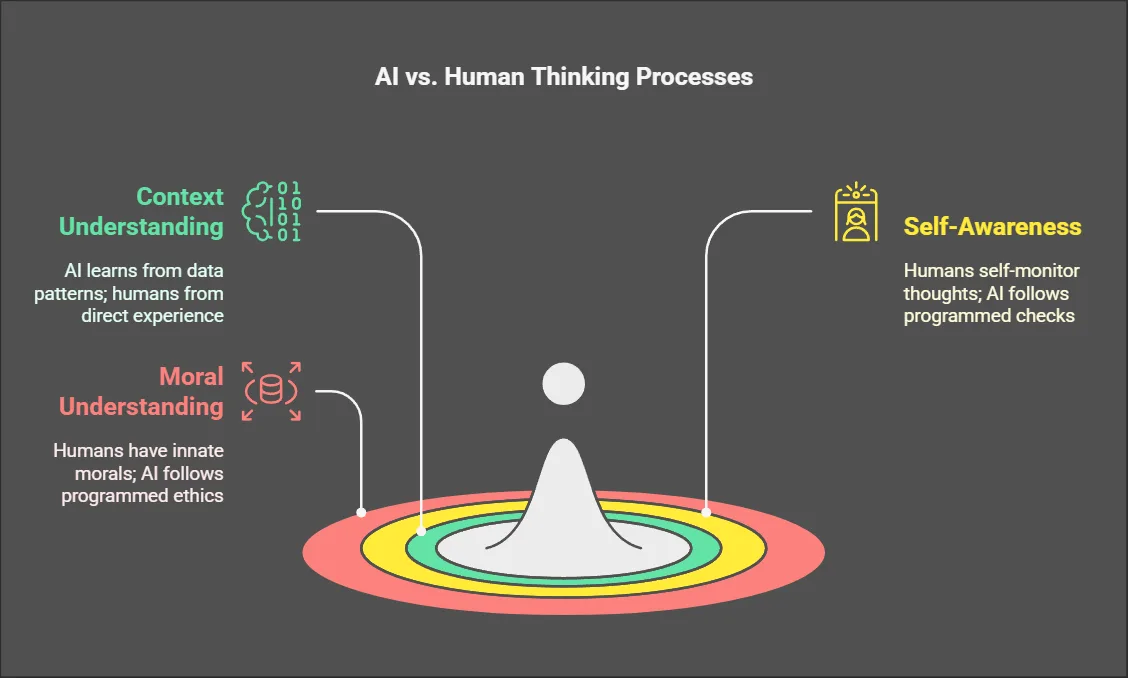
The Global Impact of AI Reasoning Models
Economic and Business Effects
The development of these advanced AI reasoning models has created major waves in the technology industry. When DeepSeek announced their dramatically lower prices for using R1 ($0.0003 per 1,000 tokens compared to OpenAI’s $0.005), it caused significant market changes. Nvidia, a leading company in AI hardware, saw its market value drop by $600 billion as investors realized future AI systems might not need as much expensive hardware.
This cost reduction is changing how businesses think about using AI. Small companies that couldn’t afford advanced AI systems before can now access powerful reasoning capabilities. This democratization of AI technology is leading to innovation across many industries, from healthcare to education.
A New Phase in AI Development
The relationship between computing power and AI capability is changing. DeepSeek’s R1 shows that smarter programming can achieve better results with less computing power. While OpenAI’s o1 needs 28.7 petaFLOP-days (a measure of computing power) to train, R1 only needs 19.4 petaFLOP-days to achieve similar results.
This efficiency improvement is particularly important because it shows that advances in AI don’t always require more powerful computers. Instead, clever programming and better understanding of how AI learns can lead to significant improvements.
International Competition and Cooperation
The development of these AI reasoning models has intensified the technology race between different countries, particularly the United States and China. This competition has led to some interesting trends
The movement of AI researchers between companies has increased, with about 14% of OpenAI’s research staff moving to open-source projects since early 2025. This shift shows how the field of AI development is becoming more diverse and less centralized.
Different regions are taking different approaches to regulating AI. The European Union is working on specific laws for AI reasoning models, while the United States focuses on controlling the export of AI-related hardware. These different approaches create challenges for companies working across multiple countries.
The Science Behind AI Reasoning
Combining Different Types of Intelligence
Modern AI reasoning models use a combination of approaches to solve problems. They mix traditional neural networks (good at recognizing patterns) with symbolic logic (good at following strict rules). This combination helps the AI work more like human experts who use both intuition and formal knowledge to solve problems.
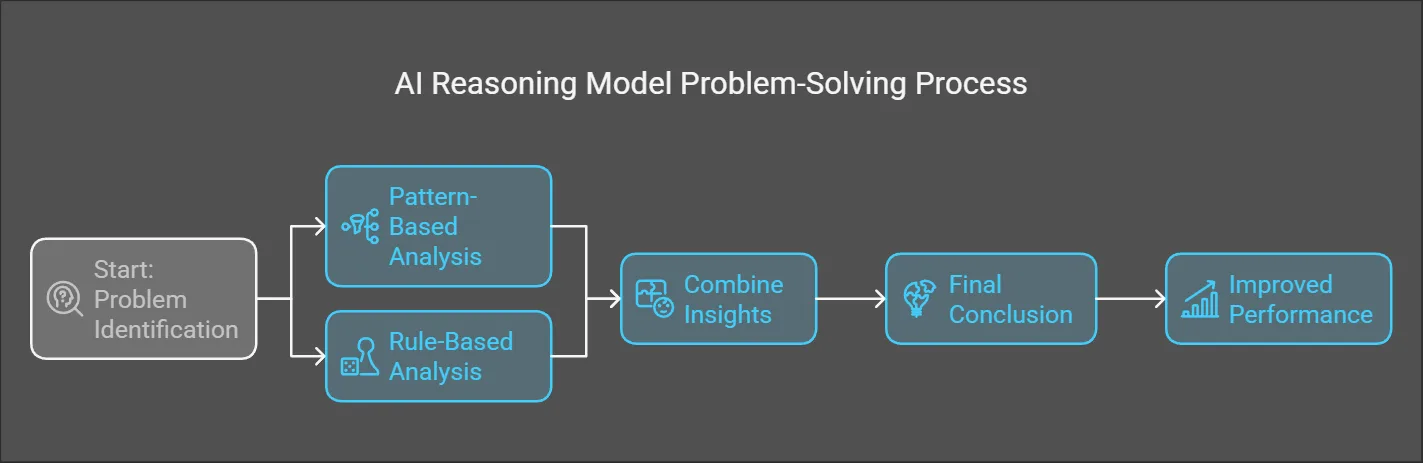
The formula that describes this approach looks complex but works in a straightforward way: the AI considers both pattern-based answers and rule-based answers, then combines them to reach a final conclusion. This method helps the AI perform 37% better on new types of problems it hasn’t seen before.
Practical Applications and Real-World Results
The real-world impact of these AI reasoning models is already visible in several areas.
Medical Diagnosis: AI systems based on R1 are showing impressive results in healthcare. They’re detecting cancer 22% more accurately than human radiologists, potentially saving many lives through earlier diagnosis.
Legal Analysis: Both o1 and R1 can understand and analyze legal documents with high accuracy, making legal research faster and more thorough. This capability helps lawyers work more efficiently and could make legal services more accessible to more people.
Research and Development: These AI models are helping scientists process and understand complex research data, potentially speeding up the development of new medicines and technologies.
Looking to the Future
Short-Term Predictions (2025-2027)
Experts have made several predictions about how AI reasoning models will develop in the next few years.
By late 2026, we might see AI systems that can outperform top human experts in at least three different specialized fields. This development could change how we think about expertise and professional services.
The cost of training advanced AI models is expected to continue dropping. By 2027, it might cost less than $1 million to create a model with human-level reasoning abilities in specific areas. This cost reduction could lead to many more organizations developing their own AI systems.
Legal challenges about AI responsibility and rights are likely to reach major courts by 2026. These cases will help establish important rules about who is responsible when AI systems make mistakes or cause problems.
Challenges and Considerations
While these developments are exciting, they also raise important concerns that need to be addressed.
Safety and Control
As AI reasoning models become more powerful and widely available, ensuring they’re used safely becomes more important. This includes preventing misuse for harmful purposes like creating sophisticated fake news or cyber attacks.
Job Market Changes
As AI systems become better at complex reasoning tasks, some professional jobs might change significantly. This could require many workers to learn new skills or change how they work.
Ethical Considerations
As AI systems take on more complex decision-making roles, we need to ensure they understand and respect human values and ethical principles.
Recommendations for the Future of AI Reasoning
Guidelines for Different Groups
Technology Companies and Developers
The rapid advancement of AI reasoning models creates both opportunities and responsibilities for technology companies. Companies working with these technologies should focus on making their AI systems more transparent and accountable. This means clearly documenting how their AI makes decisions and implementing strong safety measures.
Developers should consider using hybrid systems that combine neural networks with traditional logic systems. This approach helps create AI that can explain its reasoning process and catch potential errors before they cause problems. Companies should also invest in systems that can detect and prevent potential misuse of their AI technology.
Government and Policy Makers
Governments face the challenge of supporting AI innovation while protecting public interests. Clear regulations are needed for AI reasoning models, particularly in sensitive areas like healthcare and finance. Policy makers should work on creating “Reasoning Transparency Standards” that require companies to explain how their AI systems make decisions.
International cooperation is also crucial. Countries need to work together to prevent an AI arms race while ensuring the benefits of AI reasoning models are shared globally. This might include creating international agreements about how computing resources for AI should be allocated and used.
Educational Institutions
Schools and universities need to prepare students for a world where AI reasoning models are common tools. This means:
- Teaching students to work effectively with AI systems while maintaining their own critical thinking skills
- Updating curricula to include understanding of AI capabilities and limitations
- Focusing on skills that complement AI rather than compete with it
Making AI Work for Society
As AI reasoning models become more powerful, ensuring they benefit society becomes increasingly important. One suggested approach is creating “AI Cooperatives” – organizations where the public has a say in how AI systems are developed and used. These cooperatives could help ensure AI development aligns with public interests rather than just commercial goals.
Companies developing AI systems should implement reward systems that discourage harmful behaviors. This means programming AI to value beneficial outcomes for society, not just achieving specific tasks efficiently. They should also build in safeguards against potential misuse or unintended consequences.
While AI reasoning models become more capable, maintaining meaningful human control remains crucial. This includes developing better ways for humans to understand and interact with AI systems. Some experts suggest investing in new interfaces that help humans and AI work together more effectively.
Organizations should establish clear processes for human oversight of AI decisions, especially in critical areas like healthcare or financial services. This ensures AI remains a tool to enhance human capabilities rather than replace human judgment entirely.
The Path Forward
Balancing Innovation and Safety
The development of AI reasoning models like o1 and R1 shows both the potential and challenges of advancing AI technology. While these systems offer tremendous benefits – from improved medical diagnosis to more efficient legal analysis – they also require careful management to prevent misuse.
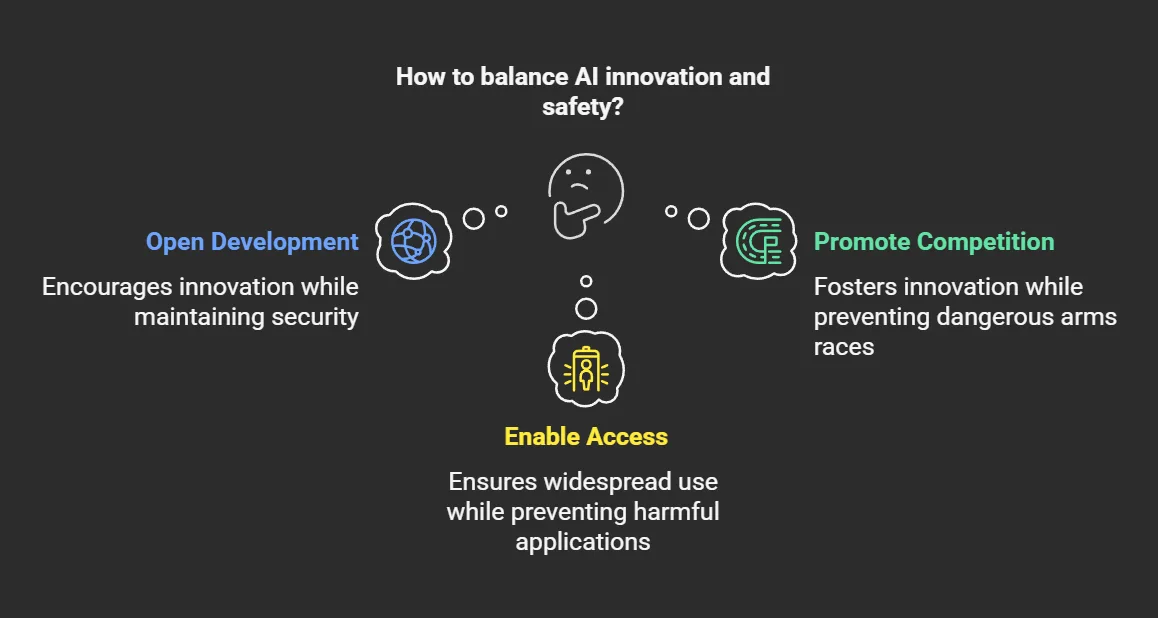
The key is finding the right balance between encouraging innovation and ensuring safety. This means:
- Supporting open development while maintaining security
- Promoting competition while preventing dangerous arms races
- Enabling widespread access while preventing harmful applications
Future Opportunities
The continuing development of AI reasoning models opens up exciting possibilities across many fields:
- Healthcare: More accurate diagnosis and personalized treatment plans
- Scientific Research: Faster discovery of new materials and medicines
- Education: Personalized learning experiences adapted to each student
- Business: More efficient decision-making and problem-solving tools
Challenges to Address
As these technologies continue to develop, several challenges need attention:
- Ensuring Fair Access: Making sure the benefits of AI reasoning models are available to everyone, not just wealthy organizations
- Protecting Privacy: Developing ways to train AI systems while protecting personal data
- Managing Economic Changes: Helping workers and businesses adapt to AI-driven changes in the job market
Conclusion
The development of AI reasoning models like OpenAI’s o1 and DeepSeek’s R1 marks a significant milestone in artificial intelligence. These systems demonstrate that AI can match human expert performance in many areas while being more accessible and affordable than ever before.
However, this progress brings both opportunities and responsibilities. The success of these technologies will depend not just on technical advancement, but on how well we manage their development and implementation. This includes creating appropriate regulations, ensuring fair access, and maintaining human oversight.
As we move forward, collaboration between technology companies, governments, and the public will be crucial. By working together, we can harness the potential of AI reasoning models while addressing the challenges they present. The goal should be to create AI systems that enhance human capabilities and contribute positively to society.
The future of AI reasoning models looks promising, but it requires careful guidance and thoughtful implementation. With proper management and continued innovation, these technologies can help solve some of our most pressing challenges while creating new opportunities for human advancement and understanding.
How does DeepSeek’s R1 model compare to OpenAI’s o1 in terms of performance?
DeepSeek’s R1 model demonstrates comparable performance to OpenAI’s o1 across various specialized domains. In legal reasoning, R1 achieves 89.7% accuracy compared to o1’s 92.3%, both exceeding average human expert performance of 88.1%. Similar patterns emerge in other fields, with R1 showing strong results in quantum chemistry (91.4% vs o1’s 94.1%) and mathematical proofs (97.5% vs o1’s 98.2%). While o1 maintains a slight edge in raw performance metrics, R1’s ability to achieve these results with significantly lower computational resources represents a major breakthrough in AI efficiency.
What are the main differences between DeepSeek’s approach and OpenAI’s approach to AI reasoning?
The key difference lies in their architectural approaches. DeepSeek employs a Sparse Mixture-of-Experts architecture that activates only 20-30% of neural pathways for each task, significantly reducing computational overhead. They also implement a hybrid symbolic-neural execution system that combines pattern recognition with formal logic engines. In contrast, OpenAI’s o1 uses a more traditional dense transformer architecture with separate System 1 (fast, intuitive) and System 2 (slow, deliberative) processing components. OpenAI focuses on recursive verification loops and dynamic computation graphs, allocating varying compute resources based on task complexity.
How does the cost of using DeepSeek’s R1 model impact its adoption compared to OpenAI’s o1?
The cost difference between the two models is substantial and has significant implications for adoption. DeepSeek offers R1 at $0.0003 per 1,000 tokens, while OpenAI charges $0.005 per 1,000 tokens for o1 – a 94% cost reduction. This dramatic price difference has led to widespread adoption of R1, with over 17,400 commercial applications integrating R1 APIs within just 72 hours of its release. The lower cost barrier has particularly benefited smaller businesses and developers who previously couldn’t afford advanced AI capabilities, leading to a surge in AI-powered applications across various sectors.
What are the potential benefits of DeepSeek’s R1 model for everyday users?
R1’s open-source nature and lower cost structure create several benefits for everyday users:
1. More affordable access to advanced AI capabilities through cheaper applications and services
2. Greater variety of AI-powered tools, as demonstrated by the surge in productivity applications on the Apple App Store
3. Improved medical diagnostic tools, with R1-based systems showing 22% higher cancer detection rates than human radiologists.
4. Access to sophisticated legal analysis and research tools that were previously available only to large organizations
5. Enhanced educational resources through AI-powered learning platforms that can adapt to individual learning styles
How does the reasoning process of DeepSeek’s R1 model differ from that of OpenAI’s o1?
The reasoning processes of these models differ in several key aspects. R1 uses a hybrid approach that combines neural pattern recognition with formal logic engines, allowing it to verify reasoning steps in real-time and catch 68% of potential hallucination errors before generating final outputs. The model optimizes learning through reinforcement from process feedback, making parameter updates 4.7 times more efficient than traditional methods.
OpenAI’s o1, on the other hand, uses a sequential approach that separates fast intuitive processing from slower deliberative reasoning. It allocates 83% more computational resources to critical inference points and employs multiple solution paths with cross-checking mechanisms. While both models achieve similar end results, R1’s approach prioritizes efficiency and real-time verification, while o1 focuses on thorough recursive verification and dynamic resource allocation.