Artificial Intelligence is reshaping the global technological landscape, influencing industries such as healthcare, finance, logistics, and beyond. This unprecedented growth in AI adoption comes with an urgent need for robust security practices. Gartner projects that over 80% of enterprises will utilize Generative AI applications by 2026. This rapid evolution creates a dual-edged reality: while AI systems enhance efficiency, they also expose organizations to novel security risks.
Cybersecurity professionals must rise to this challenge by mastering AI security. This skill goes beyond traditional approaches, demanding a deep understanding of machine learning, bias mitigation, compliance, and emerging attack vectors. Securing AI systems is no longer optional; it is the cornerstone of modern cybersecurity.
The Evolving Threat Landscape in AI Systems
AI’s transformative potential also makes it an attractive target for cyberattacks. Unlike traditional software systems, AI introduces unique vulnerabilities. These include the dependency on massive datasets, the dynamic behavior of machine learning algorithms, and the complexity of their underlying architectures. Attackers are constantly innovating ways to exploit these weaknesses, creating an evolving threat landscape.
AI-specific cyber threats have moved far beyond theoretical scenarios. Adversarial attacks, where carefully manipulated inputs deceive AI systems, are now a reality. For example, a slightly altered image can mislead facial recognition software into making incorrect identifications. Similarly, data poisoning—where attackers inject corrupted data into training datasets—can significantly undermine the reliability of AI models.
Cybercriminals are also deploying sophisticated techniques to extract sensitive information from AI models. Model inversion attacks allow attackers to reverse-engineer a model’s logic, while membership inference attacks expose whether specific data points were included in training. These vulnerabilities highlight the urgent need for specialized security measures tailored to AI systems.
Why Machine Learning Is Critical to AI Security
Securing AI systems starts with understanding their foundations. Machine learning forms the backbone of most AI applications, enabling systems to process data, recognize patterns, and make decisions autonomously. However, this reliance on data-driven learning introduces new challenges.
Unlike traditional software, which operates based on predefined rules, machine learning models adapt and evolve. This dynamic behavior makes them highly effective but also prone to errors and manipulation. For instance, overfitting—a common issue in supervised learning—occurs when a model becomes too tailored to its training data, reducing its ability to generalize to new inputs. Attackers often exploit these weaknesses to compromise the integrity of AI systems.
Professionals venturing into AI security must build a strong understanding of machine learning concepts, including supervised and unsupervised learning, reinforcement learning, and neural networks. They should also familiarize themselves with advanced techniques such as feature engineering and deep learning. These foundational skills are critical for identifying and mitigating vulnerabilities in AI systems.
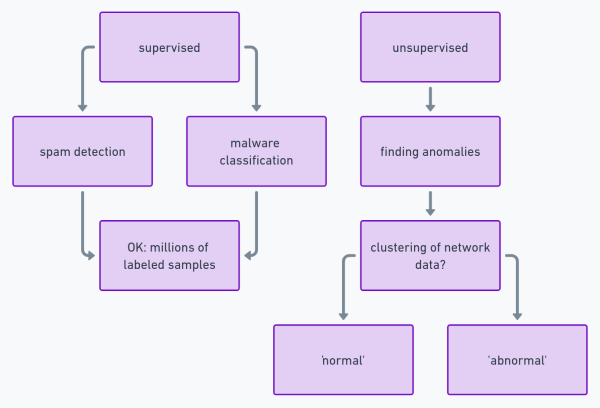
Machine learning forms the foundation of AI, making its understanding essential for security professionals:
- Healthcare AI Example: A cancer detection AI trained predominantly on images from one demographic may misdiagnose patients from underrepresented groups, reducing its accuracy and fairness.
- Adversarial Input Example: Attackers modify a tumor image slightly to bypass detection, highlighting the need to strengthen model defenses.
- Overfitting Example: A fraud detection AI model that performs perfectly on training data but fails in real-world scenarios due to over-specialization.
The Ethical Imperative of Mitigating Bias in AI
Bias in AI systems is more than a technical flaw—it is an ethical and security concern. Biased AI models can lead to discriminatory outcomes, undermining trust and exposing organizations to legal liabilities. For example, an AI-powered hiring system biased against certain demographics can reinforce existing inequities while creating new vulnerabilities.
The sources of bias in AI systems are diverse. Sampling bias occurs when training data does not represent the full diversity of real-world scenarios, while algorithmic bias arises from the design of machine learning models themselves. Measurement bias, which results from flawed data collection methods, can further compound these issues.
Mitigating bias requires a proactive approach. Organizations must invest in diverse and representative datasets, implement rigorous validation protocols, and adopt techniques such as adversarial training. Ethical AI practices not only enhance fairness but also strengthen the resilience of AI systems against manipulation.
Bias in AI has tangible real-world consequences:
- Recruitment AI Example: An AI tool penalizes resumes with terms like “women’s chess club” while favoring male-coded terms, perpetuating workplace gender disparities.
- Facial Recognition Bias Example: Systems trained only on light-skinned individuals fail to accurately identify people with darker skin tones, leading to unjust outcomes.
- Mitigation Example: Techniques like re-sampling datasets or adversarial training are used to correct biases and improve fairness.
Navigating the Regulatory Landscape
The regulatory environment surrounding AI is rapidly evolving. Governments and international bodies are introducing new frameworks to ensure the responsible and secure use of AI technologies. These regulations aim to address ethical, technical, and operational challenges, creating a complex compliance landscape for organizations.
The EU AI Act is a landmark regulation that categorizes AI systems based on their risk levels. High-risk applications, such as those used in healthcare or law enforcement, face stringent security and ethical requirements. Similarly, the NIST AI Risk Management Framework provides comprehensive guidance for managing AI risks, emphasizing transparency, accountability, and robustness.
Organizations must also navigate global data protection laws, such as the General Data Protection Regulation (GDPR) and the California Consumer Privacy Act (CCPA). These laws impose strict requirements on the handling of personal data, which is often integral to AI systems. Compliance is not only a legal necessity but also a strategic opportunity to build trust with stakeholders.
Developing Robust Risk Management Strategies
AI security goes beyond technical safeguards; it requires a holistic approach to risk management. Effective strategies integrate governance, assessment, and response mechanisms to address the full spectrum of AI-related risks.
Governance frameworks provide the foundation for responsible AI practices. They establish policies, define accountability, and set ethical guidelines for AI development and deployment. Risk assessment methodologies, meanwhile, focus on identifying potential threats and evaluating their impact. Threat modeling, which maps out possible attack vectors, is particularly valuable for understanding how vulnerabilities might be exploited.
Incident response is another critical component of AI risk management. Organizations must develop detailed playbooks for responding to breaches, including strategies for containment, investigation, and recovery. Regular drills and simulations can help teams prepare for real-world scenarios, minimizing downtime and damage.
AI Security as a Defensive and Offensive Tool
AI is not only a target for attackers but also a powerful weapon in the fight against cybercrime. AI-powered tools can analyze vast amounts of data, detect anomalies, and respond to threats in real-time. For instance, behavioral analytics systems use machine learning to identify unusual patterns in user activity, flagging potential breaches before they escalate.
Threat intelligence platforms leverage AI to predict emerging risks by analyzing data from multiple sources. These systems can identify new malware strains, phishing campaigns, and other threats, providing organizations with actionable insights. AI is also transforming incident automation, enabling faster and more efficient responses to security events.
However, the dual-use nature of AI poses challenges. Cybercriminals are increasingly using AI to automate attacks, creating sophisticated malware and phishing campaigns. This arms race underscores the need for cybersecurity professionals to stay ahead of adversaries by continually updating their skills and tools.
Emerging Trends in AI Security
The future of AI security is shaped by rapid advancements in technology and evolving threat landscapes. One of the most promising developments is Explainable AI (XAI), which aims to make AI systems more transparent. By providing clear explanations for their decisions, XAI enhances trust and facilitates the identification of vulnerabilities.
Federated learning is another emerging trend that addresses privacy concerns. This technique enables AI models to learn collaboratively without sharing raw data, reducing the risk of data breaches. As organizations adopt federated learning, they must implement security measures to protect the integrity of distributed systems.
Quantum computing represents both a challenge and an opportunity for AI security. While quantum computers have the potential to break traditional encryption methods, they also offer new possibilities for securing AI systems. Preparing for this paradigm shift is essential for staying ahead in the cybersecurity field.
Becoming an AI Security Expert
Mastering AI security requires a combination of technical knowledge, ethical awareness, and strategic thinking. Professionals must stay informed about emerging threats, technologies, and regulations, while continuously refining their skills. Networking with peers, attending industry events, and participating in training programs are valuable ways to stay ahead in this dynamic field.
AI security is more than a career path—it is a mission to protect the technologies shaping the future. By investing in this skill, cybersecurity professionals can make a meaningful impact, safeguarding organizations and individuals from the risks of an increasingly AI-driven world.